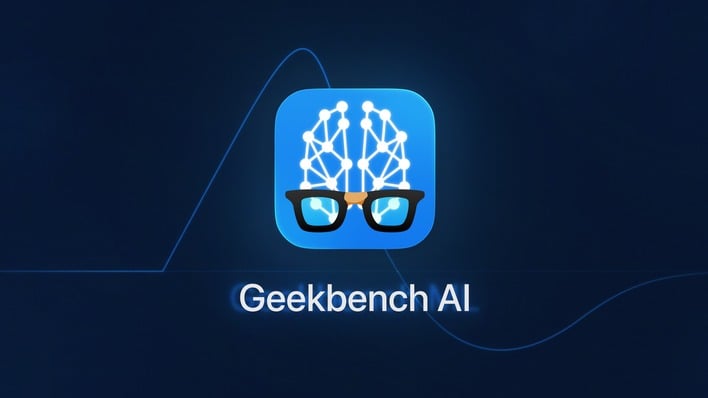
As Primate Labs points out, AI compute tasks are rather unique as workloads, meaning that they present performance characteristics that really don’t line up with any other type of benchmark. Seeking to serve the need for easy-to-run, standardized, cross-platform AI benchmarks, the Geekbench developer has just released version 1.0 of its simply-named Geekbench AI benchmark.
Geekbench AI runs ten separate tests, eight of which fall into the domain of Computer Vision while the remaining two are Natural Language Processing benchmarks. Scores are based not only on how rapidly the machine or component can iterate on the test but also on how accurate the output is compared to a “ground truth” result obtained using full-precision processing.

The benchmark application itself will look familiar to anyone who has ever used Geekbench, but there are a few selections to make before you start the benchmark this time. You see, not only do you need to pick what AI framework you’re benchmarking, but you also need to select what AI backend you’re going to use, and this affects which devices you can benchmark.
For example, on the author’s Razer Blade 18 laptop, he can use the ONNX framework to test either the Intel Core i9-14900HX CPU or, with DirectML, the machine’s GeForce RTX 4090 Laptop GPU. Alternatively, we have the option of using OpenVINO to test the CPU or the integrated Intel GPU. What we can’t do is test the NVIDIA GPU using OpenVINO, as it isn’t supported.
Just like with the standard Geekbench test, the free version requires an internet connection, as your “price” for the system test is that you must upload your results to the Geekbench browser for all to see. Above, you can see a result from the GeForce RTX 4090 in our Blade 18 laptop, and you’ll notice that there are three separate benchmark results. This is because AI workloads are commonly run with various different amounts of quantization.
We’ve cropped the result for the screenshot, but as with other Geekbench tests, you can scroll down and see the individual benchmark results. The Geekbench AI database, even in its infancy as it is, provides the ability to simply see the latest results or to sort by the highest results in each category. Here are what are currently the top results in the database for each type:

The fastest FP32 results, predictably, all come from massive discrete GPUs, although somewhat surprisingly, the highest score to date comes from a Radeon RX 7900 XT — not even the top-end “XTX” model. Going down from there we see a succession of smaller and slower GPUs, culminating in the slowest result from the first page, an NVIDIA RTX A6000 professional GPU that uses the same GPU core as the enthusiast-focused RTX 3080 just above.

The fastest half-precision results are likewise from discrete GPUs, although it seems like NVIDIA dominates here. Despite the results shifting around a bit, the same specimens are represented here. But what about in the “Quantized” results?

Well, that’s a bit different! Whether due to the QNN library being drastically more optimized than ONNX or DirectML, because Intel and AMD GPUs don’t handle the “quantized” format very well, or perhaps simply due to Qualcomm’s dedicated NPU being that fast, the top results in the quantized tests are all relatively low-power mobile processors. It’s a fascinating dichotomy, and one that we’d love to dive deeper into if we were AI developers.
We aren’t, though, so hopefully someone with more expertise provides some deeper insights into why these benchmark results look the way we do. If you’re interested in testing your own machine’s AI prowess, head to the Primate Labs site to snag Geekbench AI 1.0; it’s available for Windows, Linux, macOS, iOS, and Android, for both x86-64 and ARM devices.