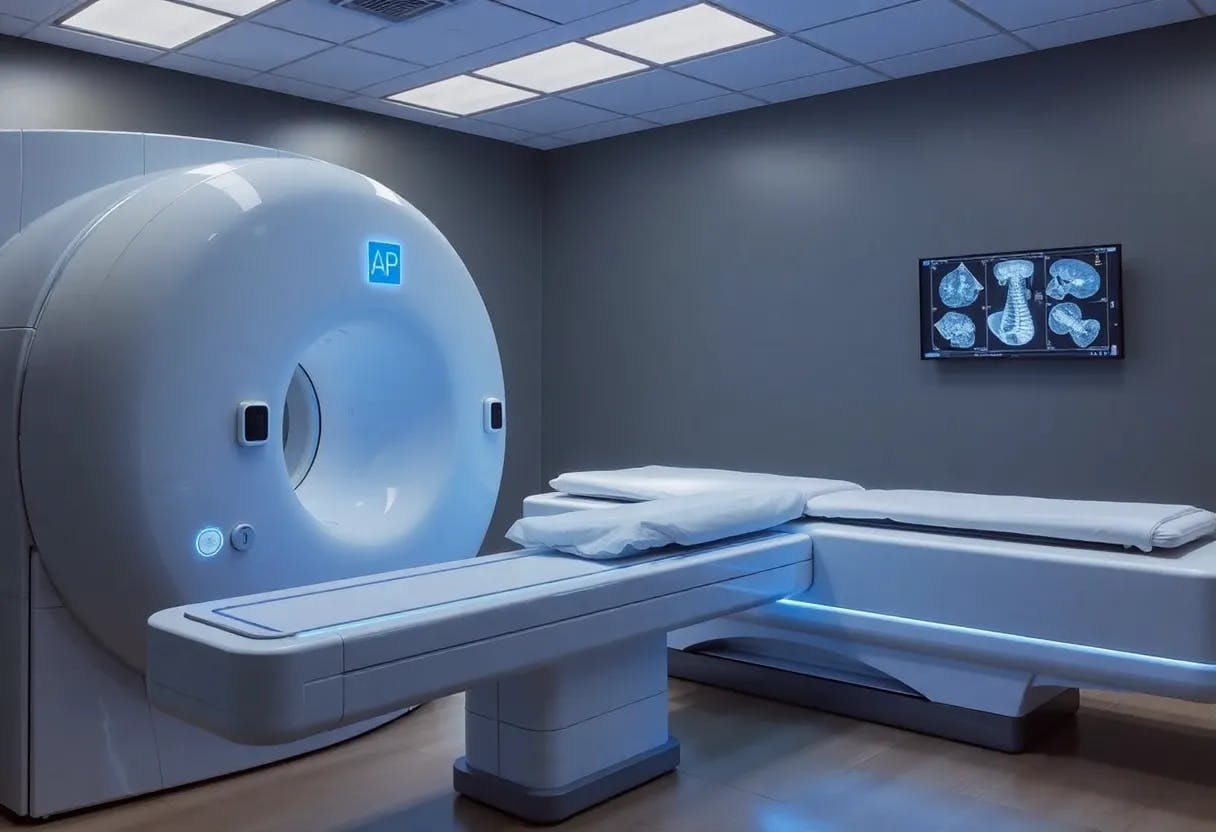
Research Suggests AI Models Can Deliver More Accurate Diagnoses Without Discrimination | HackerNoon
In this paper, we presented the notion of positive-sum fairness and argued that larger disparities are not necessarily harmful, as long as it does not come at the expense of a specific subgroup performance. The general performance, standard fairness and positive-sum fairness of four models was analyzed, each leveraging sensitive attributes in a different way. Our study highlights the need for a nuanced understanding of fairness metrics and their implications in real-world applications. Good incorporation