Listen to this article |
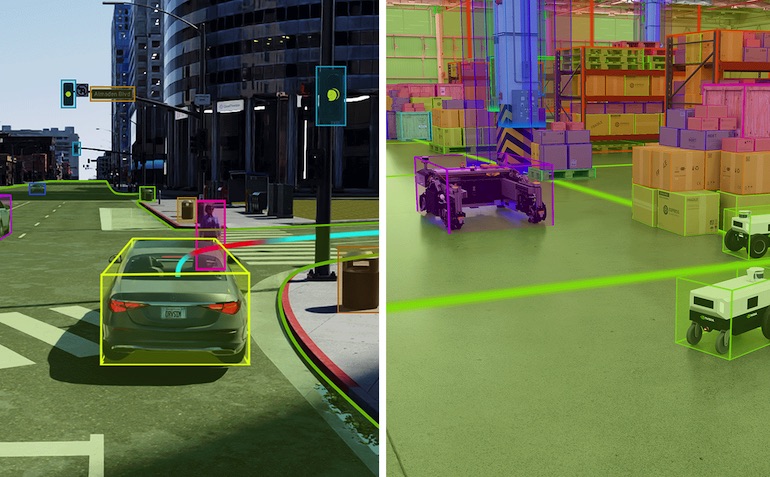
As shown at CVPR, Omniverse Cloud Sensor RTX microservices generate high-fidelity sensor simulation from
an autonomous vehicle (left) and an autonomous mobile robot (right). Sources: NVIDIA, Fraunhofer IML (right)
NVIDIA Corp. today announced NVIDIA Omniverse Cloud Sensor RTX, a set of microservices that enable physically accurate sensor simulation to accelerate the development of all kinds of autonomous machines.
NVIDIA researchers are also presenting 50 research projects around visual generative AI at the Computer Vision and Pattern Recognition, or CVPR, conference this week in Seattle. They include new techniques to create and interpret images, videos, and 3D environments. In addition, the company said it has created its largest indoor synthetic dataset with Omniverse for CVPR’s AI City Challenge.
Sensors provide industrial manipulators, mobile robots, autonomous vehicles, humanoids, and smart spaces with the data they need to comprehend the physical world and make informed decisions.
NVIDIA said developers can use Omniverse Cloud Sensor RTX to test sensor perception and associated AI software in physically accurate, realistic virtual environments before real-world deployment. This can enhance safety while saving time and costs, it said.
“Developing safe and reliable autonomous machines powered by generative physical AI requires training and testing in physically based virtual worlds,” stated Rev Lebaredian, vice president of Omniverse and simulation technology at NVIDIA. “Omniverse Cloud Sensor RTX microservices will enable developers to easily build large-scale digital twins of factories, cities and even Earth — helping accelerate the next wave of AI.”
Omniverse Cloud Sensor RTX supports simulation at scale
Built on the OpenUSD framework and powered by NVIDIA RTX ray-tracing and neural-rendering technologies, Omniverse Cloud Sensor RTX combines real-world data from videos, cameras, radar, and lidar with synthetic data.
Omniverse Cloud Sensor RTX includes software application programming interfaces (APIs) to accelerate the development of autonomous machines for any industry, NVIDIA said.
Even for scenarios with limited real-world data, the microservices can simulate a broad range of activities, claimed the company. It cited examples such as whether a robotic arm is operating correctly, an airport luggage carousel is functional, a tree branch is blocking a roadway, a factory conveyor belt is in motion, or a robot or person is nearby.
Microservice to be available for AV development
CARLA, Foretellix, and MathWorks are among the first software developers with access to Omniverse Cloud Sensor RTX for autonomous vehicles (AVs). The microservices will also enable sensor makers to validate and integrate digital twins of their systems in virtual environments, reducing the time needed for physical prototyping, said NVIDIA.
Omniverse Cloud Sensor RTX will be generally available later this year. NVIDIA noted that its announcement coincided with its first-place win at the Autonomous Grand Challenge for End-to-End Driving at Scale at CVPR.
The NVIDIA researchers’ winning workflow can be replicated in high-fidelity simulated environments with Omniverse Cloud Sensor RTX. Developers can use it to test self-driving scenarios in physically accurate environments before deploying AVs in the real world, said the company.
Two of NVIDIA’s papers — one on the training dynamics of diffusion models and another on high-definition maps for autonomous vehicles — are finalists for the Best Paper Awards at CVPR.
The company also said its win for the End-to-End Driving at Scale track demonstrates its use of generative AI for comprehensive self-driving models. The winning submission outperformed more than 450 entries worldwide and received CVPR’s Innovation Award.
Collectively, the work introduces artificial intelligence models that could accelerate the training of robots for manufacturing, enable artists to more quickly realize their visions, and help healthcare workers process radiology reports.
“Artificial intelligence — and generative AI in particular — represents a pivotal technological advancement,” said Jan Kautz, vice president of learning and perception research at NVIDIA. “At CVPR, NVIDIA Research is sharing how we’re pushing the boundaries of what’s possible — from powerful image-generation models that could supercharge professional creators to autonomous driving software that could help enable next-generation self-driving cars.”
Foundation model eases object pose estimation
NVIDIA researchers at CVPR are also presenting FoundationPose, a foundation model for object pose estimation and tracking that can be instantly applied to new objects during inference, without the need for fine tuning. The model uses either a small set of reference images or a 3D representation of an object to understand its shape. It set a new record on a benchmark for object pose estimation.
FoundationPose can then identify and track how that object moves and rotates in 3D across a video, even in poor lighting conditions or complex scenes with visual obstructions, explained NVIDIA.
Industrial robots could use FoundationPose to identify and track the objects they interact with. Augmented reality (AR) applications could also use it with AI to overlay visuals on a live scene.
NeRFDeformer transforms data from a single image
NVIDIA’s research includes a text-to-image model that can be customized to depict a specific object or character, a new model for object-pose estimation, a technique to edit neural radiance fields (NeRFs), and a visual language model that can understand memes. Additional papers introduce domain-specific innovations for industries including automotive, healthcare, and robotics.
A NeRF is an AI model that can render a 3D scene based on a series of 2D images taken from different positions in the environment. In robotics, NeRFs can generate immersive 3D renders of complex real-world scenes, such as a cluttered room or a construction site.
However, to make any changes, developers would need to manually define how the scene has transformed — or remake the NeRF entirely.
Researchers from the University of Illinois Urbana-Champaign and NVIDIA have simplified the process with NeRFDeformer. The method can transform an existing NeRF using a single RGB-D image, which is a combination of a normal photo and a depth map that captures how far each object in a scene is from the camera.
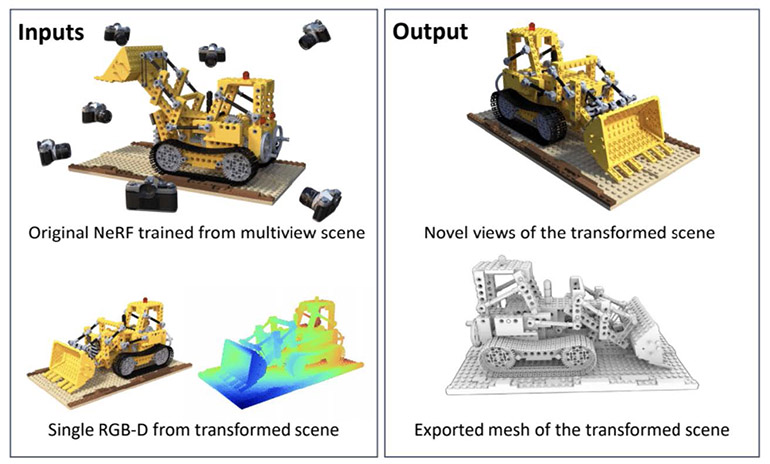
Researchers have simplified the process of generating a 3D scene from 2D images using NeRFs. Source: NVIDIA
JeDi model shows how to simplify image creation at CVPR
Creators typically use diffusion models to generate specific images based on text prompts. Prior research focused on the user training a model on a custom dataset, but the fine-tuning process can be time-consuming and inaccessible to general users, said NVIDIA.
JeDi, a paper by researchers from Johns Hopkins University, Toyota Technological Institute at Chicago, and NVIDIA, proposes a new technique that allows users to personalize the output of a diffusion model within a couple of seconds using reference images. The team found that the model outperforms existing methods.
NVIDIA added that JeDi can be combined with retrieval-augmented generation, or RAG, to generate visuals specific to a database, such as a brand’s product catalog.
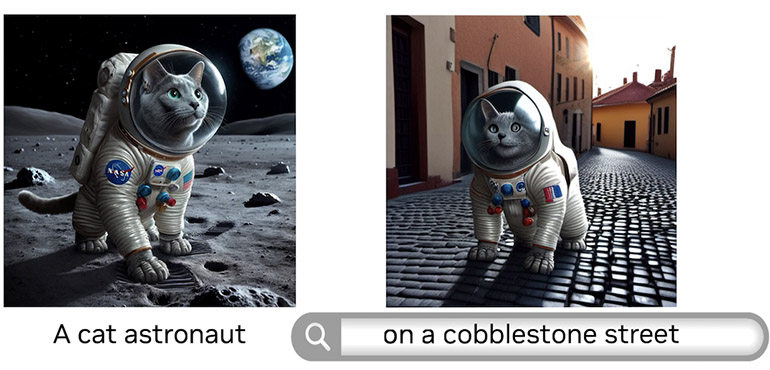
JeDi is a new technique that allows users to easily personalize the output of a diffusion model within a couple of seconds using reference images, like an astronaut cat that can be placed in different environments. Source: NVIDIA
Visual language model helps AI get the picture
NVIDIA said it has collaborated with the Massachusetts Institute of Technology (MIT) to advance the state of the art for vision language models, which are generative AI models that can process videos, images, and text. The partners developed VILA, a family of open-source visual language models that they said outperforms prior neural networks on benchmarks that test how well AI models answer questions about images.
VILA’s pretraining process provided enhanced world knowledge, stronger in-context learning, and the ability to reason across multiple images, claimed the MIT and NVIDIA team.
The VILA model family can be optimized for inference using the NVIDIA TensorRT-LLM open-source library and can be deployed on NVIDIA GPUs in data centers, workstations, and edge devices.
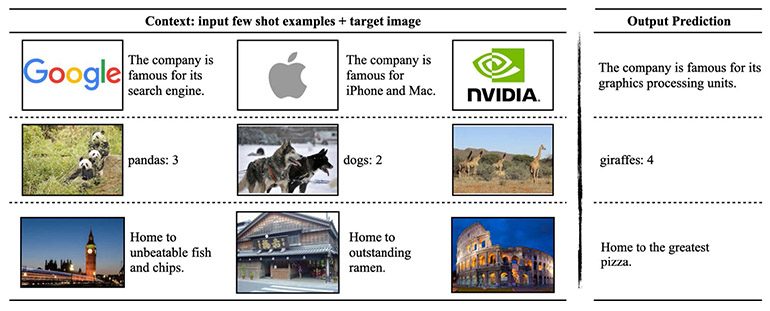
VILA can understand memes and reason based on multiple images or video frames. Source: NVIDIA
Generative AI drives AV, smart city research at CVPR
NVIDIA Research has hundreds of scientists and engineers worldwide, with teams focused on topics including AI, computer graphics, computer vision, self-driving cars, and robotics. A dozen of the NVIDIA-authored CVPR papers focus on autonomous vehicle research.
“Producing and Leveraging Online Map Uncertainty in Trajectory Prediction,” a paper authored by researchers from the University of Toronto and NVIDIA, has been selected as one of 24 finalists for CVPR’s best paper award.
In addition, Sanja Fidler, vice president of AI research at NVIDIA, will present on vision language models at the Workshop on Autonomous Driving today.
NVIDIA has contributed to the CVPR AI City Challenge for the eighth consecutive year to help advance research and development for smart cities and industrial automation. The challenge’s datasets were generated using NVIDIA Omniverse, a platform of APIs, software development kits (SDKs), and services for building applications and workflows based on Universal Scene Description (OpenUSD).
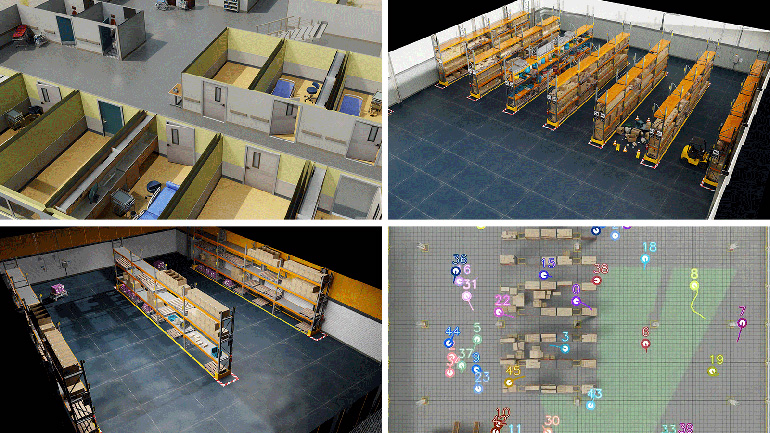
AI City Challenge synthetic datasets span multiple environments generated by NVIDIA Omniverse, allowing hundreds of teams to test AI models in physical settings such as retail and warehouse environments to enhance operational efficiency. Source: NVIDIA
About the author
Isha Salian writes about deep learning, science and healthcare, among other topics, as part of NVIDIA’s corporate communications team. She first joined the company as an intern in summer 2015. Isha has a journalism M.A., as well as undergraduate degrees in communication and English, from Stanford.